Valizadegan's work is just one stunning example of how AI is giving us a more detailed view of the cosmos.
瓦利扎德甘的工作只是人工智能如何让我们更详细地了解宇宙的一个令人惊叹的例子。
Only a few years ago, scientists from an international team called the Event Horizon Telescope published the first image of a black hole.
就在几年前,来自事件视界望远镜国际团队的科学家发布了第一张黑洞影像。
The effort involved hundreds of researchers stitching together data from radio telescopes placed around the world.
这项工作涉及数百名研究人员将来自世界各地电波望远镜的数据拼接在一起。
The resulting image, however monumental, appeared blurry because of the telescopes' limitations.
由此产生的影像,无论多么巨大,由于望远镜的限制而显得模糊。
A computational astrophysicist and a member of that team, Lia Medeiros built an algorithm that finds patterns in the radio data and creates a new version of the image.
计算天体物理学家兼该团队的成员莉亚·梅德罗斯建立了一种算法,可以在无线电数据中找到模式并创建新版本的图像。
The algorithm, called PRIMO, did not sharpen the image in the way a photographer might eliminate blurriness using Photoshop.
这种名为PRIMO的算法并没有像摄影师使用Photoshop消除模糊那样锐利化影像。
Rather, it put together a brand-new image, as if a Photoshop user built a fresh picture.
相反,它组合了一个全新的图像,就像Photoshop用户建立了一张新图片一样。
The result is a higher definition image than the one produced by the Event Horizon Telescope, with the black hole's features more neatly resolved.
结果是比事件视界望远镜产生的影像清晰度更高,黑洞的特征更清晰地解析。
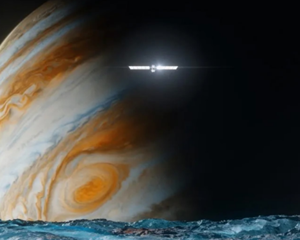
Medeiros believes PRIMO could be used to construct images of other mysterious objects.
梅德罗斯相信PRIMO可以用来建构其他神秘物体的图像。
Some of the most interesting processes of planet formation are still invisible to us, even with the best telescopes.
即使使用最好的望远镜,我们仍然看不到一些最有趣的行星形成过程。
Large radio telescopes can record the dust and gas in protoplanetary disks where planets form, and optical telescopes can see fully formed worlds, but the in-between stages of growth don't come through as clearly.
大型射电望远镜可以记录行星形成的原行星盘中的尘埃和气体,光学望远镜可以看到完全形成的世界,但中间的生长阶段并没有那么清晰。
Medeiros thinks systems like PRIMO can improve the resolution of Earth's most sensitive telescopes, perhaps bringing these mysteries into view.
梅德罗斯认为像PRIMO这样的系统可以提高地球上最灵敏的望远镜的分辨率,或许可以让人们看到这些奥秘。
Despite all the potential, Medeiros says, some scientists are still wary of machine learning. The black box problem remains.
梅德罗斯说,尽管机器学习潜力巨大,但一些科学家仍然对机器学习持谨慎态度。黑盒子问题仍然存在。
Medeiros notes she built PRIMO from scratch with transparency in mind. But not all astronomical questions can be answered with such bespoke programs.
梅德罗斯指出,她从头开始构建PRIMO时就考虑到了透明度。但并非所有天文问题都可以通过此类定制程序得到解答。
ExoMiner is more of a black box, built on existing neural networks and then heavily refined by Valizadegan and his colleagues.
ExoMiner更像是黑盒子,建立在现有的神经网络之上,然后由瓦利扎德甘和他的同事进行了大量改进。
But astronomers grew to trust it, especially once it began finding planets.
但天文学家在逐渐信任它,尤其是当它开始寻找行星时。